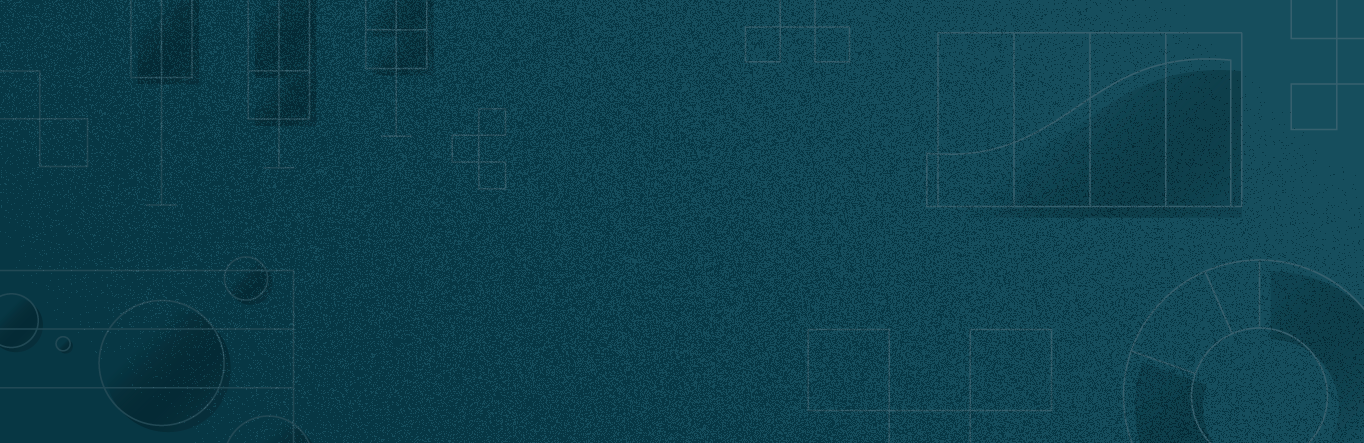
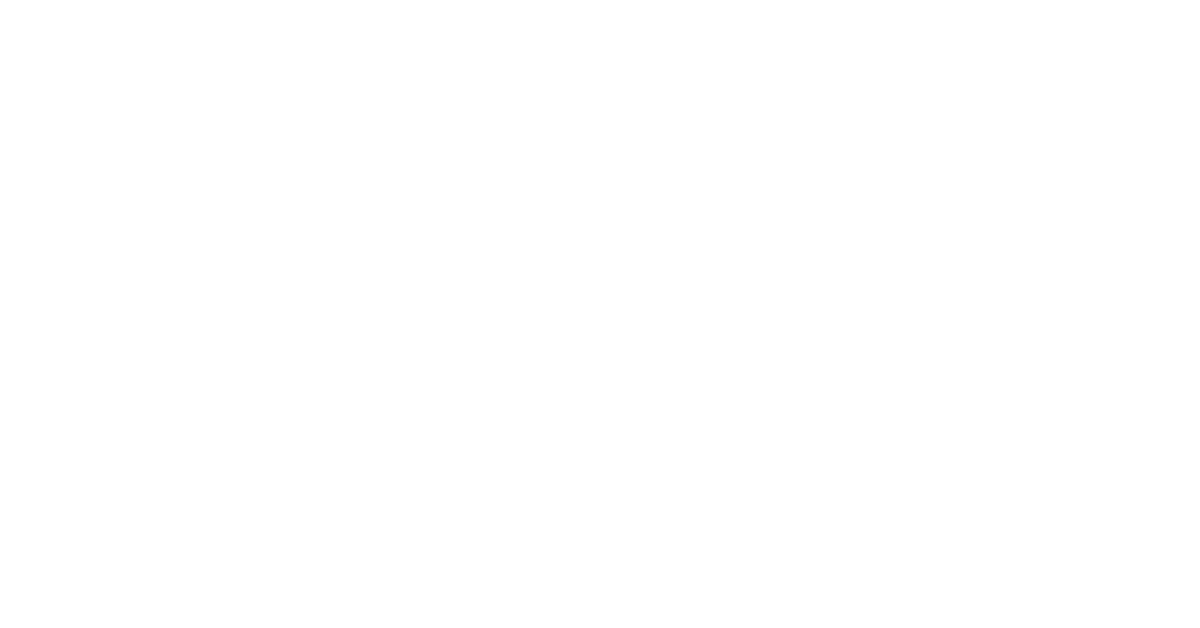
Unlocking Planning in Pharma with AI/ML
Pharma companies are leaning into the power of AI/ML to improve forecasting capabilities. And establishing a robust organizational structure, namely ensuring IT and supply chain are synced, is paramount to the success of these implementations.
Key Takeaways
-
1
When specifically looking at forecasting roles, 95% of pharma companies mention AI/ML skills as a key requirement in job posts, compared to 79% of companies across the other industries in our data set.
-
2
Our analysis of 100 B2C brands indicates that incorporating AI/ML into the planning function is correlated with higher gross margin growth, specifically three full percentage points above the median.
AI and ML will be the top technology investment for 60% of life sciences companies over the next two years, according to results from a global survey fielded by Pistoia Alliance. Despite 54% of these companies already using AI/ML, respondents know they still have work to do to ensure successful implementations – reporting data quality and poor curation are the biggest barriers they face. This is because AI/ML models are still data fragile. Successful implementation requires a stronger collaboration between business and IT functions than in previous waves of digital innovation. To address this, pharma leaders have taken to investing in AI/ML talent across all functional areas but with a particular eye on forecasting, supply planning, inventory optimization, and network strategy.
Our analysis of 100 B2C brands showed that the top ten companies that incorporated AI/ML into planning job role descriptions saw gross profit margins three percentage points above the median in our data set. Companies have caught on – as is made clear by the high levels of investment in recruiting talent with AI/ML skills – with an average of 87% of companies mentioning these specific skills in supply chain job posts across all industries.
Bayer, one of the largest pharma and biomedical companies in the world, has made strides in the AI/ML space within forecasting, leveraging data from private GenAI models and adapting to supply chain processes. BioGPT, a GenAI biomedical text generation, was initially built for product development and marketing. The tech proved hugely successful, accelerating the productivity of the medical affairs team by nearly 100x. Seeing potential, the Bayer supply chain team started using the unstructured data that came from those activities and converted it into usable forecast insights, ultimately doubling forecast accuracy.
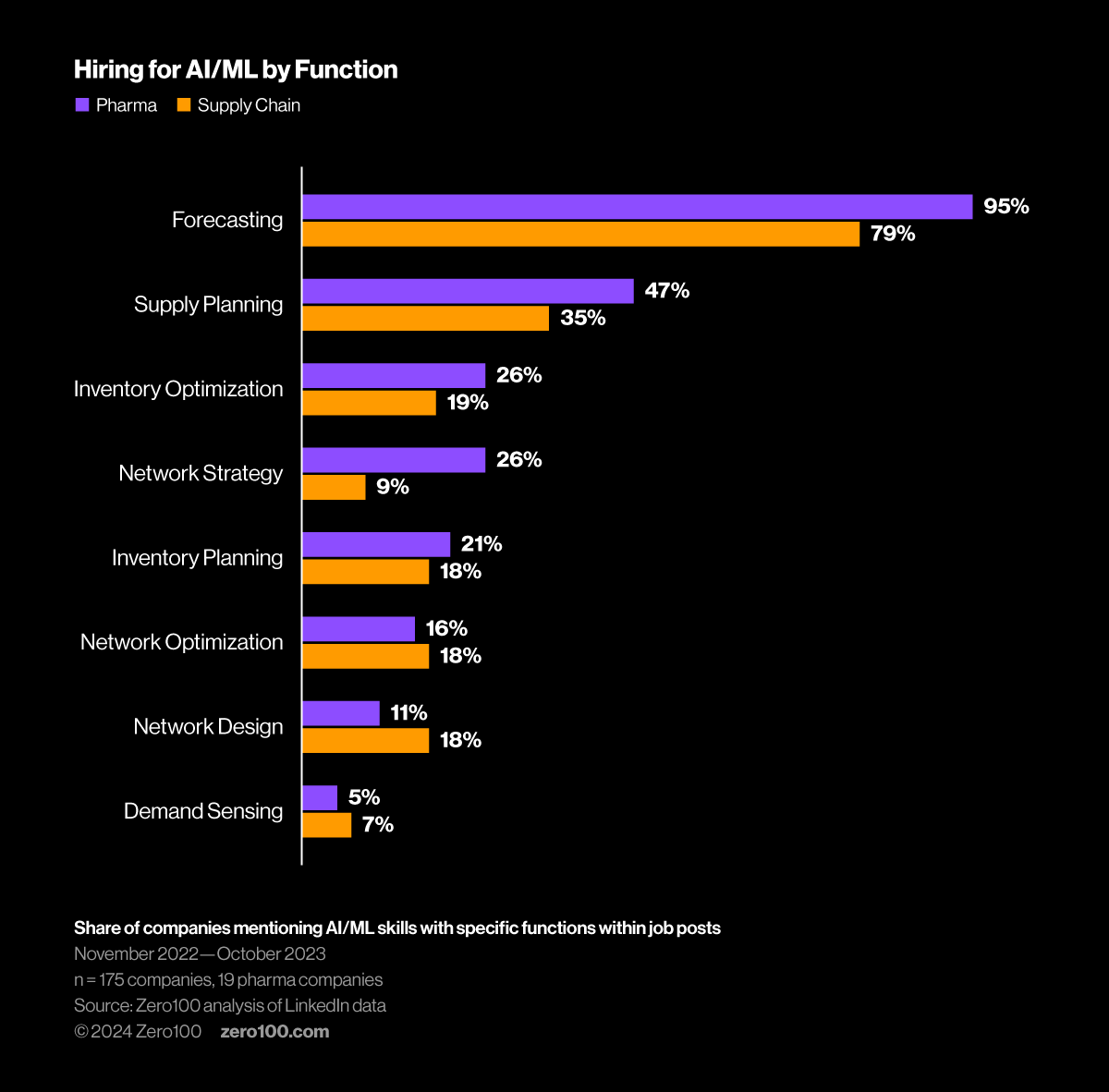
The increased incorporation of AI/ML within planning underscores the importance of establishing a robust organizational structure to manage the data. Laying this foundation starts with the integration of IT and supply chain functions. Johnson & Johnson, a pharma company leading the way on this organizational fusion, has created an intelligent supply chain by gathering data based on pre-set parameters across their supply chain, which it uses to build an analytics layer, from which they build data tools. These tools are then used in forecasting, along with other advanced planning initiatives. One use of the data is calculating the average length of time a particular product takes to travel through the end-to-end supply chain, which then allows the company to provide accurate delivery forecasts for customers.
How can you pave the way for successful AI implementations across your supply chain? Lay the foundation by setting up continuous C-level AI strategy reviews. Supply chain leaders in your organization should be taking time to think about and discuss the AI roadmap for their function with peers. As a group, your leadership team can determine how to best integrate across siloes, particularly between business and IT functions. While getting aligned on strategy and roadmap, think about the emerging architectures you need to investigate and incorporate to get AI enterprise-ready.
To see a different data cut or to dig deeper into this topic, reach out to our Head of Research Analytics, Cody Stack, at Cody.Stack@zero100.com.
Methodology
Zero100’s proprietary data and analytics are a combined effort between our data scientists and research analysts. We provide data-first insights matched with our own research-backed points of view and bring this analysis to life via real-world case examples being led by supply chain practitioners today.
For this study, we looked at 2.8 million LinkedIn job posts from 175 B2B and B2C companies. Our analysis categorizes listings based on mentions of specific digital skills within job titles and descriptions.