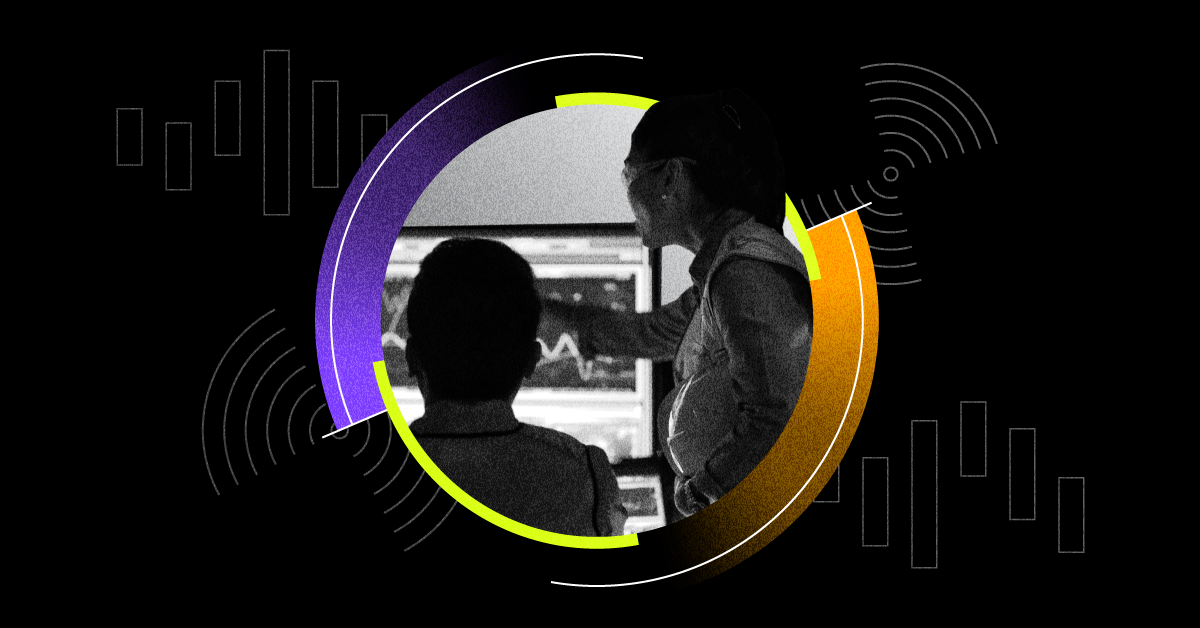
How Big Is AI for Supply Chain?
This tech revolution is different. AI is self-learning, hungry for data, and more accessible than past tech breakthroughs. But how big is AI for supply chain? It’s time to delve into bold applications, exciting results, and what it takes to turn the art of the possible into reality.
A recent New York Times article about AI told the story of Norn cells, key to the body’s ability to produce blood as needed, which were discovered in 2023 after 134 years of field research. Meanwhile, an AI model created by Stanford researchers taught itself enough developmental biology in six weeks to correctly identify the same Norn cell.
134 years of learning and discovery, replicated in six weeks with AI. What are the possibilities for supply chain?
Learning by Doing, But Much Faster
Supply chain management is a field science, meaning we invent as we go and learn by trial and error. This process is encoded in our culture as “continuous improvement” – a mindset that celebrates incremental progress on KPIs like forecast accuracy, labor productivity, and material cost. It’s been great, but it won’t create the breakthroughs needed for supply chains to be resilient and responsible enough for this bumpy twenty-first century.
Seeking breakthrough improvement on cell discovery, the Stanford team built a foundation AI model called Universal Cell Embedding (UCE) that “was trained on a corpus of cell atlas data from human and other species in a completely self-supervised way without any data annotations.” UCE’s grasp of cellular biology is so complete that it is finding clusters of function and relationships between cells for which it was not trained, potentially leading to many sudden medical breakthroughs.
Supply chains generate massive data sets from sourcing through customer use, and their performance is governed by rules inferred from experience and applied in practice. As with the UCE modeling cellular biology, supply chain works as a system, revealing its secrets through experiential learning.
AI for supply chain is big because it can accelerate our trial-and-error learning process thousand-fold.
The Art of the Possible
Judging by the buzz from Davos this past February and the fact that 44% of the 150 companies we analyzed cited AI on their Q1 2024 earnings calls, CEOs clearly expect breakthroughs, not just continuous improvement. A few public stories have fueled this fire:
- Amazon saw an immediate 1500 bp improvement in forecast accuracy using neural networks for slow-moving items.
- Lenovo cut PC production planning from six hours to 90 seconds with AI.
- Walmart conducts 2,000 supplier negotiations simultaneously with a chatbot, which most suppliers prefer to human buyers.
- Pokka, a leading beverage company in Singapore, used AI to map the carbon footprint of 4,980 SKUs in just under 100 minutes with 92% accuracy.
More generally, Zero100 data on AI leadership, as reflected in the precision of language in functional job descriptions, shows that from 2019-2022, AI leaders, on average, grew revenue 60% faster, protected margins 157 bp better, and surprised investors with actual EPS variance only one third that of laggards. They are also 15% ahead on achieving their Scope 3 carbon goals.
AI is not just another technology. It is an uber-tech turbocharging everything we do with the traditional levers of continuous improvement: people, process, and technology. We believe AI is as big as railroads, electric power, or even the internet itself, with similar potential to change everything we know about business and operations.
The Science of the Practical
Comparisons with electric power or the internet are fair, but AI is moving faster – ChatGPT took two months to get to 100M users vs Amazon, which took 12 years to reach the same number. It is also different from previous tech revolutions because it is self-learning, voraciously hungry for and facile with data, and more accessible than past tech breakthroughs.
The first practical step for most CSCOs is to get a clear picture of where AI is already running in the business, including customer-facing systems. Such an “AI census” is the basis for a CEO-friendly one-pager on your plan for AI in supply chain. Equally important is articulating the talent ecosystem you will need to drive this transformation and run it into the future. Talent 2030 looks very different from talent 2020, and it’s a problem with a long lead time.
The art of the possible imagines breakthroughs at the system level. The science of the practical starts with a clear understanding of the as-is situation and drives progress with the best bets and the brightest teams.
AI for supply chain will shortcut decades of incremental learning at a critical moment in history.
It is huge.